Key takeaways
-
Our researchers developed new methods for craniosynostosis care.
-
They created AI tools and statistical models to evaluate 3D photogrammetry.
-
3D photogrammetry is safer and less invasive than traditional imaging.
-
The tools improve accuracy for diagnosis and enable post-surgical evaluation.
Background: improving evaluation of craniosynostosis using 3D photogrammetry
Pediatric craniofacial medicine is moving away from using CT scans and MRIs, which use ionizing radiation or require sedation, to evaluate head development anomalies in favor of 3D photogrammetry. 3D photogrammetry technology uses multiple cameras to produce a three-dimensional (3D) image.
Applications for use in pediatric craniofacial medicine include:
- Quantifying craniofacial anomalies
- Planning and evaluating craniofacial surgery
- Repeated assessments of post-surgical outcomes over time
While 3D photogrammetry is a popular and safe imaging alternative, existing imaging analysis methods were not designed to operate on unstructured image data representations.
A multidisciplinary team of researchers at Children’s Hospital Colorado developed and tested a new method based on geometric deep learning that uses 3D photogrammetry to:
- Identify craniofacial landmarks in the original data structure in real time
- Automatically evaluate head shape anomalies of patients and their improvements after surgical treatment
Deep learning is a type of machine learning (artificial intelligence) that trains computational neural networks using large datasets to perform tasks fast and accurately.
In this report, study authors present a fully-automated pipeline for the quantification of post-surgical changes in children with craniosynostosis, a condition where sutures in the skull close prematurely during fetal development. Researchers included Antonio Porras, PhD; Brooke French, MD; Connor Elkhill; Jiawei Liu, MS; and David Khechoyan, MD.
Quantitative evaluation of craniosynostosis
Targeted anthropometric metrics have been proposed, such as:
- Cephalic index
- Cranial vault asymmetry index
- Metopic index
- Inter-frontal angle
Novelty of geometric deep learning using 3D photogrammetry
In this report, study authors presented:
- A novel geometric deep learning architecture that aggregates local multi-resolution features from 3D photogrammetry to detect craniofacial landmarks in real time
- Accurate metrics of head shape anomalies that enable the objective evaluation of head shape anomalies and craniosynostosis using non-invasive 3D photogrammetry
Methods: testing new methods for detection, analysis of craniosynostosis
Retrospective image datasets
Three retrospective image datasets were collected for this study. All three had specific image resolutions and slice thicknesses; there is no patient overlap among these independent datasets.
Dataset A: 3D photogrammetry
Total of 982 3D photograms
- 331 female
- 661 male
- 3.53 ± 2.35 years old
Conditions represented:
- 63.4% craniosynostosis
- 18.6% deformational plagiocephaly
- 5.1% cleft lip or palate
- 12.8% craniofacial anomalies
Timing of images
- 166 before corrective surgery
- 114 after surgery
- 314 no surgical dates
Annotation and grouping
- Each 3D photogram annotated with series of 27 craniofacial landmarks
- Bookstein classification for grouping based on ease of consistent identification within 3D photogrammetry
- Type I – easy
- Type II – challenging
- Type II – very difficult without physical examination
Dataset B: normative CT images
- Established a normative statistical reference of head anatomy for quantifying anomalies
- Consisted of retrospective CT images ordered post-trauma
- All images from individuals with clinically confirmed absence of cranial anomalies
- Additional details found in previous studies
Dataset C: CT Images of craniosynostosis patients
- Served as an independent reference to interpret head anomalies from Dataset A
- Included images from patients with various types of craniosynostosis and different fused sutures
Multi-resolution graph convolutional network for craniofacial landmark regression
The proposed novel geometric convolutional neural network used Chebyshev polynomials to:
- Exploit point connectivity information in 3D photogrammetry
- Quantify multi-resolution spatial features
Study authors then designed a landmark-specific trainable scheme that:
- Aggregates multi-resolution geometric and texture features quantified at every vertex of a 3D photogram
- Uses new probabilistic distance regression module to weigh node coordinates based on estimated probabilities to regress landmark locations
Quantification of head shape anomalies
To quantify head shape anomalies, study authors:
- Used CT images from dataset B to build sex- and age-specific variability model
- Segmented head surfaces from patient 3D photograms using cranial base landmarks detected by proposed method
- Created a new head shape anomaly index to measure independently from underlying pathology
- Trained a machine learning classifier to assess likelihood of craniosynostosis as cause of observed anomalies
Results: new framework for detection of craniofacial landmarks delivers accuracy
The experiments demonstrated a high robustness to spatial resolution variability in the 3D photograms.
The head shape anomaly index quantified a significant reduction of head shape anomalies resulting from surgical treatment:
- 1.39 ± 0.37 pre-surgical head shape anomaly index average
- 1.14 ± 0.29 post-surgical head shape anomaly index average
Discussion and conclusion: improved accuracy of craniofacial detection, surgical evaluation of craniosynostosis
Study authors concluded:
- The fully automated framework provides real-time craniofacial landmark detection from 3D photogrammetry with state-of-the-art accuracy.
- Improvements of using new landmark detection method compared to state-of-the-art methods
- Significant improvement of landmark detection performance when RGB texture and new trainable weighted aggregation scheme is used
- Better landmark detection performance when using new probabilistic regression module to identify landmark locations on 3D photogram compared to study author’s network trained without it
- Increase in robustness of new method compared to spatial resolution variability in 3D photogrammetry
- The new head shape anomaly index can quantify significant head phenotype changes and can be used to quantitatively evaluate surgical treatment in patients with craniosynostosis.
- Substantial improvements from new head shape anomaly index over previous work
- Significant reduction of head shape anomalies resulting from surgical treatment
Featured researchers

Antonio R. Porras, PhD
Research Director
Pediatric Plastic and Reconstructive Surgery
Children's Hospital Colorado
Assistant professor
Biostatistics and Informatics, Colorado School of Public Health
University of Colorado School of Medicine

Brooke French, MD, FACS
Co-director, craniofacial surgery
Center for Children's Surgery
Children's Hospital Colorado
Director of the Cosmetic Program
Associate professor of Plastic and Reconstructive Surgery
University of Colorado School of Medicine
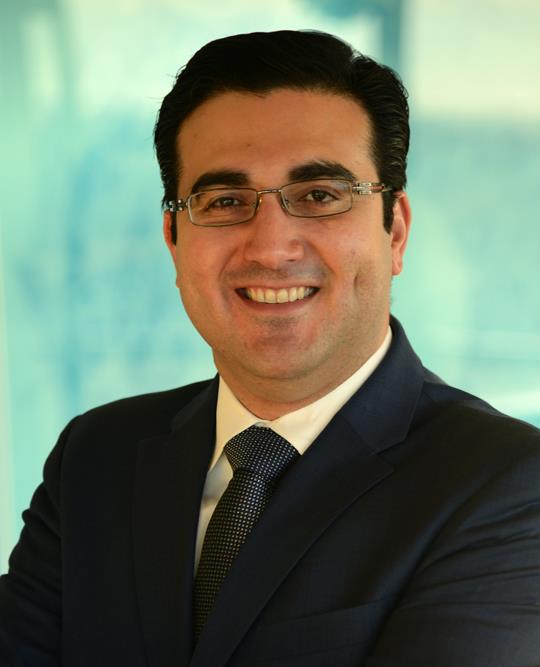
David Khechoyan, MD
Pediatric plastic and craniomaxillofacial surgeon
Plastic and Reconstructive Surgery
Children's Hospital Colorado
Associate professor
Surgery-Plastic/Reconstructive
University of Colorado School of Medicine